VelocityEHS® Operational Risk helps you identify, assess, communicate and eliminate risk to your people, property, and the environment.
Help prevent incidents before they occur with risk management software that offers a holistic view of operational risk at your organization—in one dashboard—along with the flexibility to adapt and scale with your business. Drive collaboration to prioritize and control risks across daily activities, safely manage changes in processes, and verify controls to ensure effectiveness for a safer, healthier workplace.
Explore Our Core Operational Risk Capabilities
With unparalleled visibility and control of your enterprise-wide risks, you’ll drive enhanced collaboration and engagement in your company’s global risk management activities.
Management of Change
Learn MoreHazard Studies
Learn MoreBowtie Assessments
Learn MoreRisk Analysis
Learn MoreJob Safety Analysis (JSA)
Learn MoreControl Verification
Learn MoreLibrary of Controls
Semi-Quantitative Analysis
Learn MoreManagement of Change
Learn MoreHazard Studies
Learn MoreBowtie Assessments
Learn MoreRisk Analysis
Learn MoreJob Safety Analysis (JSA)
Learn MoreControl Verification
Learn MoreLibrary of Controls
Semi-Quantitative Analysis
Learn More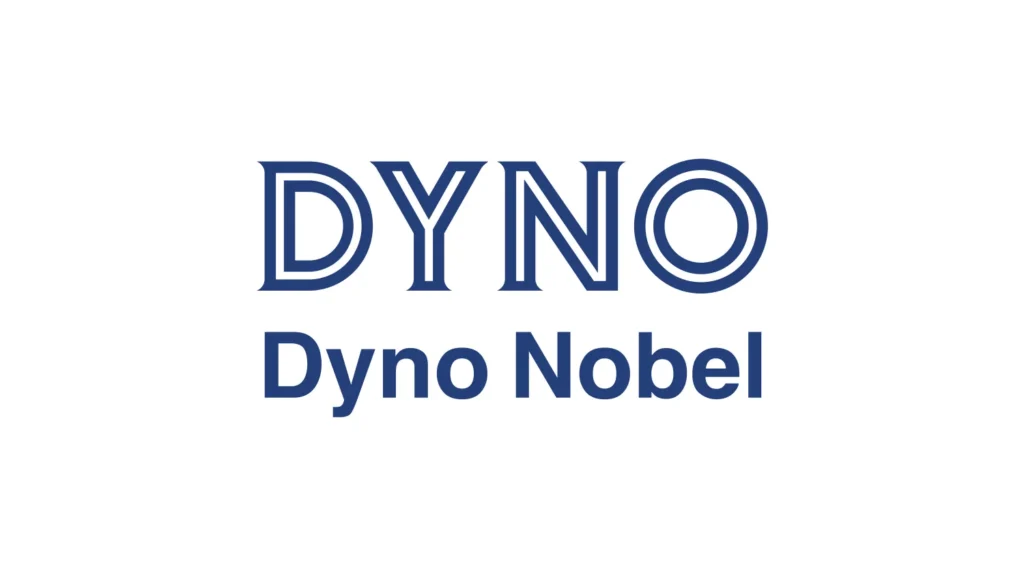
Learn how Dyno Nobel reduced risk with the help of VelocityEHS.
Case Study
Read how VelocityEHS helped this commercial explosives firm with 32 manufacturing facilities on three continents increase visibility into critical control verifications and reduce injury rates.
Benefits of VelocityEHS Operational Risk Solution
We believe that enterprise organizations deserve better. Better outcomes. Better control. Better experiences with our software solutions.
Centralize & Standardize
- Unified view of risk causes, impacts & controls
- Conduct thorough hazard analysis using flexible studies
- Easily communicate risks and critical controls with bowties
- Drive employee engagement with org-wide visibility of risks
Gain Control of Risks
- Rapidly scale risk and control programs with master bowties
- Schedule risk reviews and performance standard reviews
- Monitor risks and verify controls in real-time
- Accelerate sharing of risk management best practices
Make Risk Data Work for You
- Generate PLL, LOPA and Cost-Benefit Analysis
- Transition smoothly from qualitative to quantitative
- Provide insight on risk controls companywide
- Adhere to regulations like WHS, COMAH, OSHA PSM, ISO 45001
VelocityEHS Repeats as a Leader in Process Safety Management Software
The new Verdantix analysis reviewed 15 vendors and named Velocity as a leader for our highly capable PSM platform and “market leading” Chemical Management and Management of Change capabilities (MOC).
Learn MoreThe Accelerate Platform is
EHS & ESG done right.®
Insightful
Business intelligence & reporting
Helpful
Built-in guides & training
Friendly
3rd party Integration ready
Fast ROI
Accelerated time-to-value
Simple
All actions & tasks in one place
Informative
Auto alerts, emails & texts
Multilingual
20+ languages
Effortless
Easy to update & upgrade
Attentive
Committed customer support
Secure
GDPR & SOC 2 compliant
Learn from the experts
We’re invested in your continued education and success. Check out the latest industry news, our most recent blogs, and helpful training resources that will ensure you stay ahead of the curve.
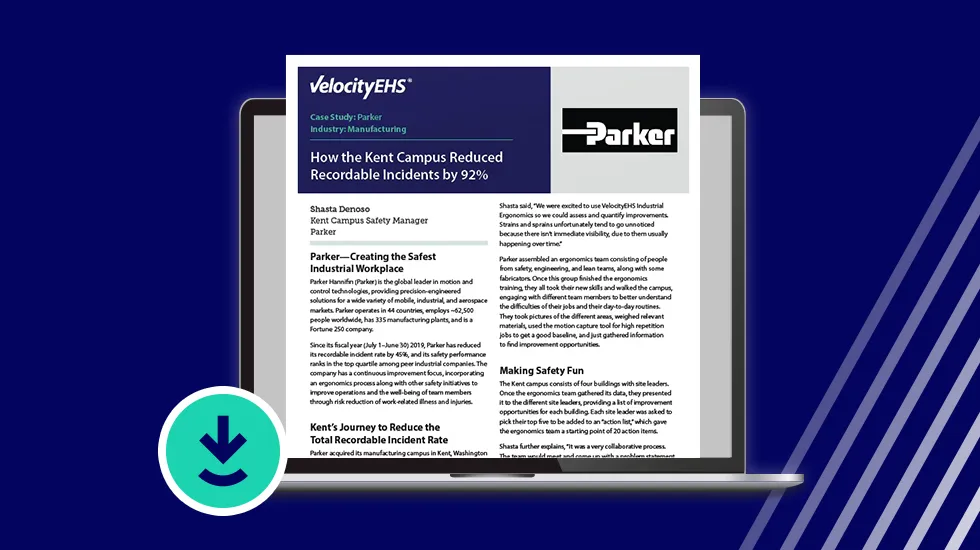
New Case Study—Parker, Kent Campus: How They Reduced Recordable Incidents by 92% Using Ergonomics
Ergonomics
Parker Kent campus shares how they made safety fun while improving employee engagement and proactively reducing workplace risks.
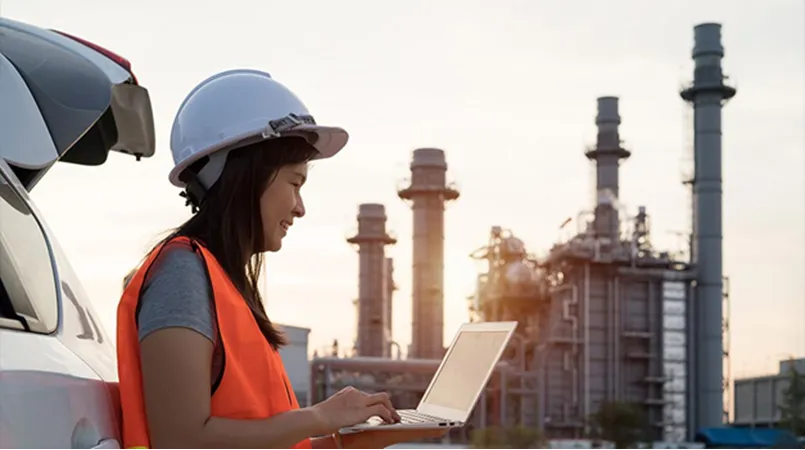
California’s ESG Disclosure Rules: What You Need to Know
ESG
California is now the first US state with ESG disclosure requirements. Here are some major takeaways about California’s ESG disclosure rules.
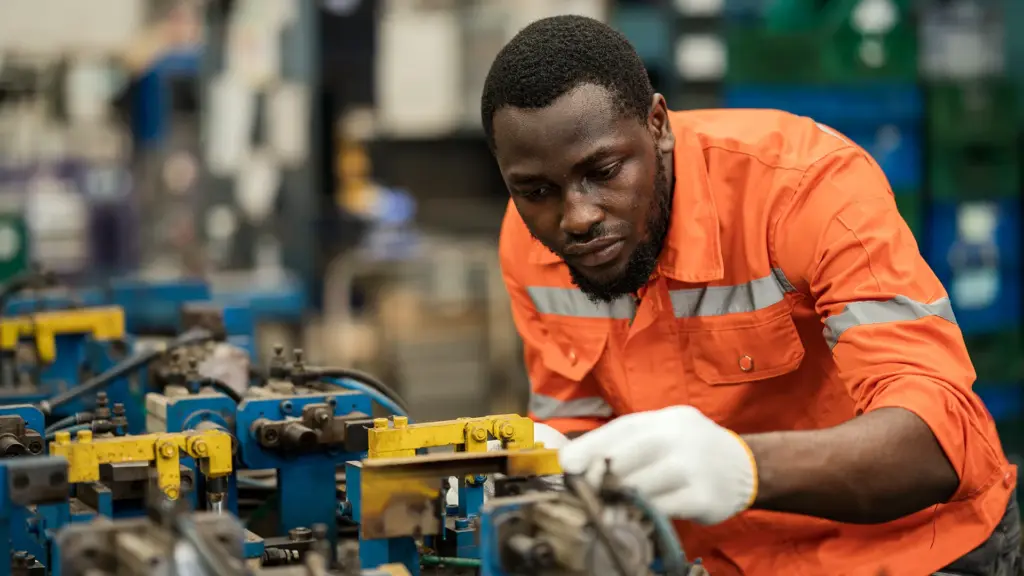
The Ergonomics Hit List®: A Simple Tool to Identify Issues in the Workplace
Ergonomics
In industrial environments, it’s important to take the time to properly assess the workplace for musculoskeletal disorder (MSDs) risks which can lead to sprains, st…
Partner with the most trusted name in the industry
Stress less and achieve more with VelocityEHS at your side. Our products and services are among the most recognized by industry associations and professionals for overall excellence and ease of use.
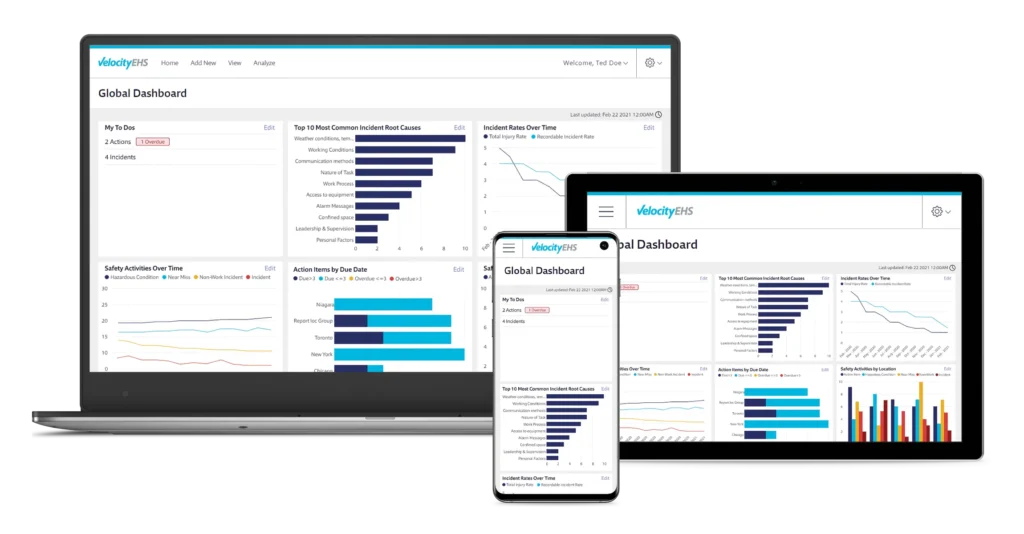
Schedule a Demo Today
You’re not just buying software or services—you’re investing in a partnership that empowers your team to proactively solve problems and move boldly into the future.